Computer-Aided Drug Design (CADD) has transformed the pharmaceutical industry by integrating computational methods into the drug discovery process. This approach enhances the efficiency, selectivity, and efficacy of drug development, significantly reducing time and costs associated with traditional methods.
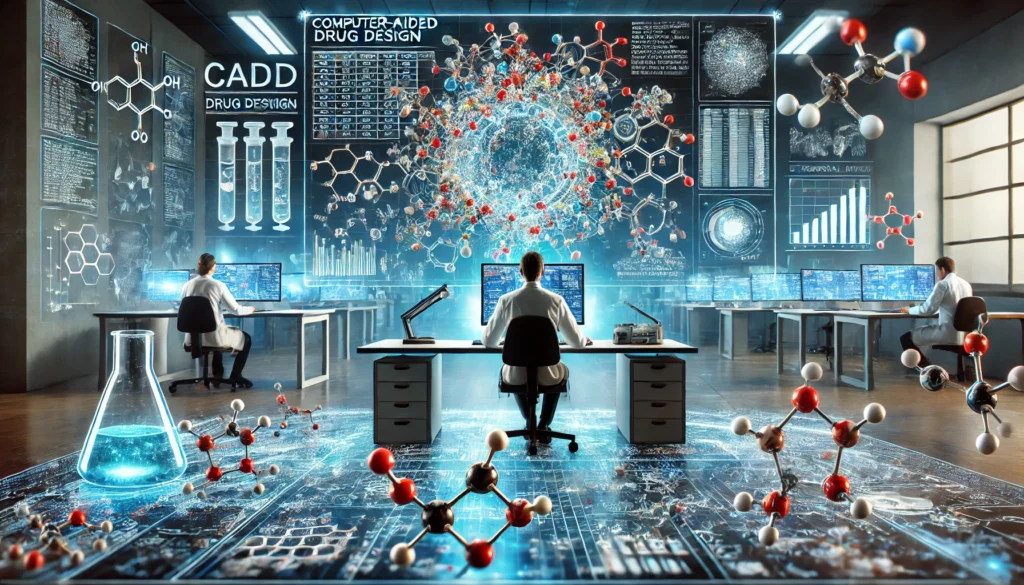
What is Computer-Aided Drug Design (CADD)?
CADD employs computational techniques to identify, optimize, and evaluate compounds that interact with biological targets. By simulating molecular interactions, CADD predicts the binding affinity and activity of potential drug candidates, facilitating the design of molecules with desired properties.
Types of CADD
- Structure-Based Drug Design (SBDD):
- Definition: Utilizes the three-dimensional structures of biological macromolecules to design drugs that specifically interact with target sites.
- Process:
- Protein Structure Determination: Acquiring high-resolution structures of target proteins through techniques like X-ray crystallography or NMR spectroscopy.
- Binding Site Identification: Locating potential binding sites on the protein surface.
- Molecular Docking: Simulating the interaction between the target and potential drug molecules to predict binding affinity.
- Virtual Screening: Assessing large compound libraries to identify promising candidates.
- Applications: Designing inhibitors for enzymes, developing receptor agonists or antagonists, and creating peptide-based drugs.
- Ligand-Based Drug Design (LBDD):
- Definition: Focuses on known ligands to develop new compounds by analyzing their chemical structures and biological activities.
- Process:
- Pharmacophore Modeling: Identifying the essential features responsible for biological activity.
- Quantitative Structure-Activity Relationship (QSAR): Correlating chemical structure with biological activity to predict the effects of new compounds.
- Virtual Screening: Searching databases for compounds that match the identified pharmacophore.
- Applications: Developing drugs when the target structure is unknown, optimizing lead compounds, and repurposing existing drugs.
Pharmacophore Modeling
Pharmacophore modeling involves creating a 3D representation of the essential features of a molecule necessary for biological activity. This model serves as a template for designing new compounds with similar activity profiles.
Lead Discovery and Optimization
The process of lead discovery and optimization includes:
- Lead Discovery: Identifying initial compounds that exhibit desired biological activity.
- Lead Optimization: Modifying chemical structures to enhance potency, selectivity, and pharmacokinetic properties.
- Strategies:
- Structure-Activity Relationship (SAR) Analysis: Assessing how changes in chemical structure affect biological activity.
- ADMET Prediction: Evaluating Absorption, Distribution, Metabolism, Excretion, and Toxicity properties to ensure drug-like characteristics.
Recent Trends in Drug Design
Advancements in technology have introduced new trends in drug design:
- Artificial Intelligence (AI) and Machine Learning: Enhancing predictive models for drug efficacy and safety.
- Quantum Computing: Potentially revolutionizing the simulation of molecular interactions.
- Green Chemistry: Focusing on sustainable and environmentally friendly drug development processes.
Applications of CADD
CADD is applied in various stages of drug development:
- Hit Identification: Using virtual screening to find compounds that interact with the target.
- Lead Optimization: Refining compounds to improve efficacy and selectivity.
- Preclinical Development: Assessing pharmacokinetics and toxicity profiles.
Limitations of CADD
Despite its advantages, CADD faces certain limitations:
- Accuracy of Predictions: Computational models may not always accurately predict biological activity.
- Complexity of Biological Systems: Simulating the full complexity of biological environments remains challenging.
- Data Quality: Dependence on high-quality, comprehensive datasets for accurate modeling.
Conclusion
Computer-Aided Drug Design has become an indispensable tool in modern pharmaceutical research, offering a systematic approach to drug discovery. By integrating computational methods with experimental data, CADD accelerates the development of effective and safe therapeutic agents. Ongoing advancements in computational power and algorithms continue to enhance the capabilities of CADD, promising a future of more efficient and personalized medicine.